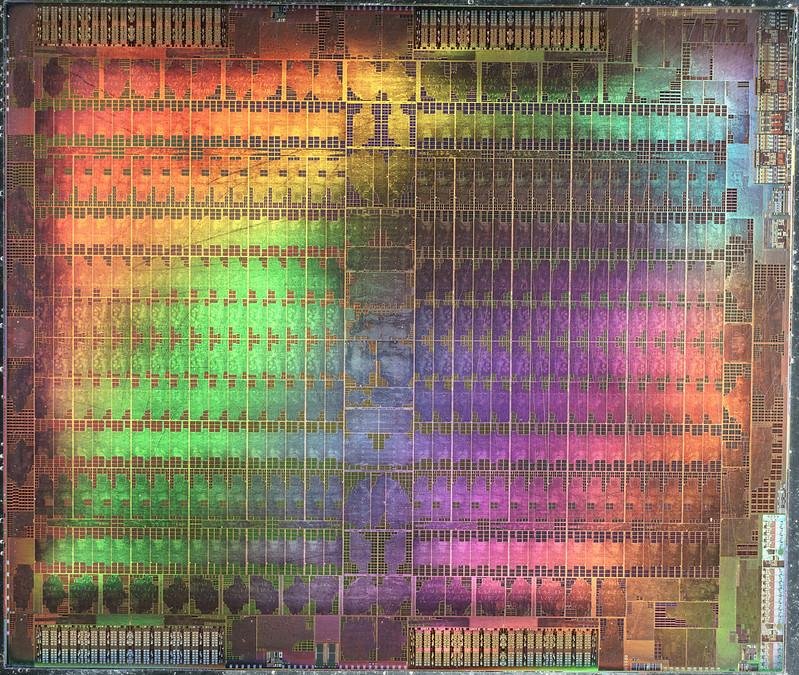
Workshop 1 : Envisioning Equitable Representation in ML Evaluation
27 April | 4.30 pm | Venue: Frankopan Hall
Facilitators: Stevie Bergman, Willie Agnew and Maribeth Rauh
Abstract: Representation in AI is intrinsically linked to value – what is represented is what is valued – and an expression of the world we want reflected in our technologies. But what does “representation” practically mean for AI systems? Similar to other terms in the responsible machine learning realm, e.g. AI “fairness” and “transparency”, we have a sense of it but the term is generally not well-defined enough to clearly operationalize best practices. We see representation in AI called for in news and opinion pieces, literature, AI guidelines, product aspirations, and proposed policy in both the EU and UK. There’s some consensus that broad representation is a net good (even necessary) element of responsible AI, yet the devil is in the details. For example, the notion that representative sampling – the creation of a set with instances proportional to that of a larger population – does not typically take into account the diversity within each group, applicability to the task at hand, or the inherently socio-political and intercultural nature of full representation. As practitioners, it is incumbent upon us to engage in participatory and justice-oriented techniques with distributed power mechanisms to allow for better representation of the diverse array of voices and needs in the design of our systems. In this workshop we will untangle notions of representation in evaluation datasets, and walk through case studies to gather hints towards best practice. Through discussion, we will uncover limitations of representation, e.g. the manners in which a mathematical or primarily technical notion of “representation” in sampling can be useful, but ultimately leave much to be desired. Together, we will engage in alternate visioning to understand what an equitable conception of representation could look like. In such a vision, how do the current practices for dataset curation and development need to change to shift power?
Author bios: Stevie (she/her) conducts sociotechnical research on DeepMind’s Ethics Research Team. She has a global, human rights focus in her work and typically investigates questions as to the impacts of technology on marginalised and in-conflict communities outside the US and Europe. Her current work is on the topics of data governance and representation, meaningful participation, and the effective alignment and evaluation of AI systems. Stevie's research often has direct implications for both ML practitioners and policy.